Conversational Analytics: A Technical Walkthrough
Who was double texting more? How long did the other person keep the other waiting for a response? Who was laughing more often? Cursing? Sharing links and articles? Emojis? which ones were most often used? Favorite curse words? Favorite way to express laughter?
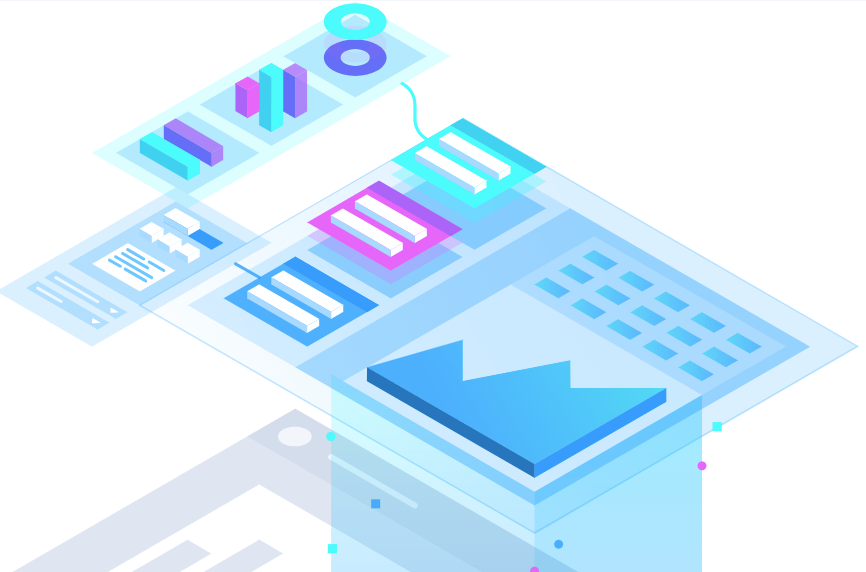